Synopsis
The increasing volume and variety of neuroimaging datasets demand advanced modeling techniques to answer scientifically relevant questions such as causality and treatment effect. This workshop features novel statistical and machine learning approaches for analyzing high-dimensional brain signals, tree-shaped sulcal and gyral structures, and neurons.
Workshop Program
- 08.00 am – 09.00 am “Modeling Spectral Causality in a Brain Network”, Hernando Ombao, King Abdullah University of Science and Technology
- 9.00 am – 10.00 am “Heat Kernel Smoothing on Riemannian Manifolds and Its Application to Brain Images”, Moo K. Chung, University of Wisconsin-Madison
- 10.00 am – 11.00 am “Using Brain Imaging for Computer-Aided Prognosis of Stroke”, Chris Rorden, University of South Carolina
- 11.00 pm – 12.00 pm “Topological Signal Processing in Neuroimaging Studies”, Yuan Wang, University of South Carolina
- 12.00 pm – 13.00 pm “Machine Learning Applied to Neuroimaging Research in Neurology”, Leonardo Bonilha, Medical University of South Carolina
- 13.00 pm – 14.00 pm “Single-Trial Identification in fMRI Data: Applications to Representation of Affective States”, Svetlana Shinkareva, University of South Carolina
- 14.00 pm – 15.00 pm “Learning with Topological Priors and Constraints”, Chao Chen, Stony Brook University
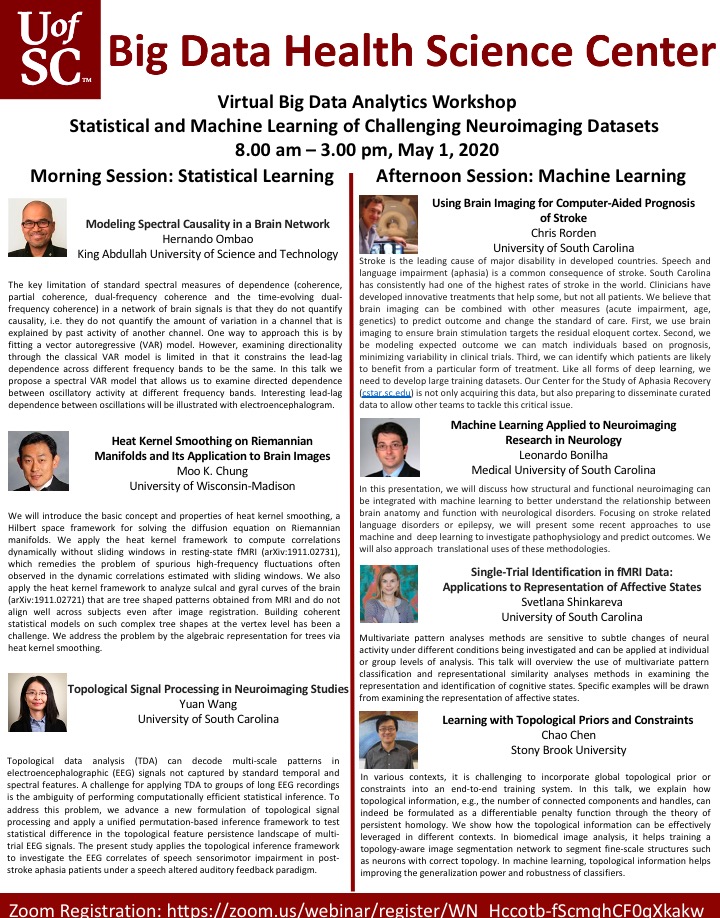