Social Media Core
About the Core
The Social Media Core aims to lead the transformation of current social science and health research at UofSC by integrating data science and social media analysis to understand health issues and human behavior. This core partners with interdisciplinary researchers across the campus and Geoinformation and Big Data Research Lab for social media applications including collection, cleaning, analysis, and visualization of large-scale social media datasets and other relevant big data. To promote interdisciplinary efforts, the core will hold hands-on workshops and speaker series to facilitate presentation, demonstration, and discussion of how social media data can be explored across a wide range of disciplines. The professional development and academic training activities will include certificates, new courses, and a new Master program in the longer term. In addition, the core will facilitate and produce collaborative manuscripts and research proposals with researchers interested in health science, data science, and geoinformatics to seek external funding opportunities from federal and other agencies. The Social Media Core is co-directed by Drs. Linwan Wu and Shan Qiao and consists of strong expertise in social media big data analytics, spatial analysis, social networks, and health disparities.
Activity 1
An increasing literature reports that a considerable proportion of COVID-19 positive people show symptoms that last for weeks or even months after recovery from acute illness (i.e., past-acute-sequela of SARS-CoV-2 [PASC]). The persistent and multi-organ, multi-system manifestations of PASC delay COVID-19 patients’ return to usual health and normal life. Building large prospective longitudinal cohorts of COVID-19 patients is a critical step to advance our understanding of PASC. In this project, we will test the feasibility of identifying COVID-19 patient cohorts using US-based Twitter data and further ascertain if we can use longitudinal information collected from this cohort for PASC symptoms analysis. Social media has a rich description of related symptoms, a longitudinal nature to allow flexible observational/analysis windows for PASC, historical information on health-related information (e.g., lifestyles and behaviors), and relatively low costs. Using Twitter data to identify a COVID-19 patient cohort could serve as a complementary and innovative strategy in data collection and analysis for investigating PASC symptoms. This study will lay important groundwork for several initiatives at the intersection of social media data mining, COVID-19 recovery, and PASC-related healthcare services.
Activity 2
Poor diet is a major contributor to the leading causes of chronic diseases and death in the United States, including coronary heart disease, diabetes, hyperlipidemia, and stroke. To better characterize and monitor US diet patterns in real-time, new sources of information need to be identified and integrated, and novel monitoring techniques need to be developed. It is essential to collect and analyze larger samples to advance knowledge and understanding of public health. Yet, traditional survey methods are expensive, difficult to deploy instantly, and fail to sample hard-to-reach populations and minorities. Social media chronicles the lives of a population, recording their beliefs, attitudes, and behaviors on a wide variety of topics. Meanwhile, the cellphone-based population mobility data records people’s visitation to different health behavior-related facilities (e.g., diet, exercise, alcohol consumption, and smoking) which can be used to measure the health risk behaviors at the population level. In this project, we explore dietary and exercise patterns on social media (i.e., Twitter data) and cellphone-based mobility data (i.e., SafeGraph) and investigate their association with health outcomes controlling for social determinants of health (SDOH) and other factors to examine health and healthcare disparities.
Activity 3
Mental disorder is a significant public health challenge in the US. It was estimated that about 30% of US adults experience a disorder once 12-month and the prevalence of lifetime disorder could be as high as 50%. Existing research and national surveillance data suggested an increase of the prevalence of mental disorders during the COVID-19 pandemic. Social media, such as Twitter, could be a source of data for prediction due to its real-time nature, high availability, and large geographical coverage. However, there is a dearth of studies on comparing accuracy of the traditional keyword-based approach and deep learning-based approach in extracting the mental health-related social media posts and validating the accuracy of Twitter-based prevalence for mental disorders through the comparison with official-reported prevalence. In this activity, we explore the feasibility of using three widely used deep learning models, including Convolutional Neural Network (CNN), Long Short-Term Memory (LSTM), and Bidirectional Encoder Representations from Transformers (BERT) to identify mental health-related (e.g., suicide) contents from the geotagged tweets in the US, and then use the extracted mental health signals to build a mental health prevalence index by validating it with the official mental health data.
Faculty
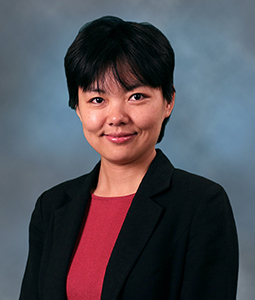
Shan Qiao, Ph.D.
Core Co-Lead
Dr. Qiao’s academic training is in sociology, anthropology and public health and her research projects use both quantitative and qualitative methodologies. Her interests are in HIV prevention and treatment in the context of global health, as well as women living with HIV/AIDS in South Carolina.
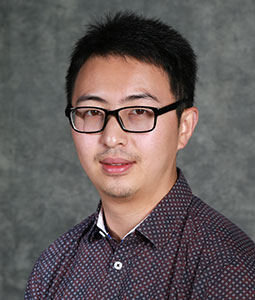
Linwan Wu, Ph.D.
Core Co-Lead
Dr. Wu’s research focuses on advertising psychology and communication technology. In general, his research attempts to understand the psychological influences of advertising messages on consumers — as well as consumers’ psychological responses to digital media and online information. Recently, his research has been dedicated to investigating consumer reactions to the integration of artificial intelligence (AI) in advertising and strategic communication. Most of Wu’s research involves conducting empirical studies using social-scientific methods.
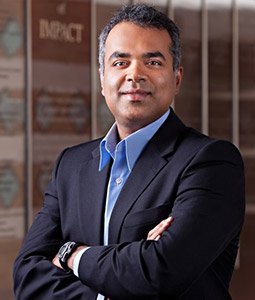
Ramkumar Janakiraman, Ph.D.
Core Member
Dr. Janakiraman’s substantive research areas include social media marketing analytics, marketing and public policy, multichannel retailing and healthcare marketing. His methodological interests include microeconometrics, applied econometric modeling and consumer learning/stuctural models. Ram’s research has appeared or forthcoming in leading marketing, information systems, operations, strategy and medical journals. Ram’s research has received several national and international research grants and recognition. Ram’s work has been covered in popular business outlets such as the Wall Street Journal and Biz Inc. He teaches graduate level courses on marketing analytics, customer relationship management, data mining and doctoral seminars on marketing models.
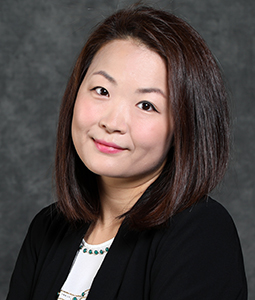
Jungmi Jun, Ph.D.
Core Member
Dr. Jun is a health communication scientist. Her research focuses on the intersection of communication/media, socio-psychology, and health perception/behavior as well as health information disparities and communicative coping of racism among racial/ethnic minorities. She applies social media analytics to surveil communication and policies surrounding novel tobacco products.
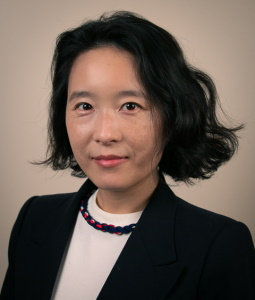
Minji Kim, Ph.D.
Core Member
Dr. Minji Kim’s research focuses on targeted and tailored health communication. Kim’s recent projects include examining the effects of targeted marketing and counter-marketing of emerging tobacco products, including e-cigarettes and heated tobacco products, with emphasis on the impact on vulnerable populations including youth and Asian Americans. Her research utilizes diverse methods including message testing experiments, qualitative interviews, and social media content analyses.
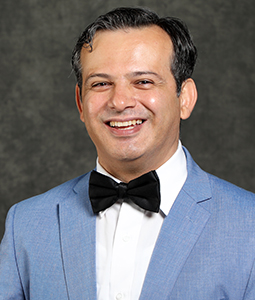
Ehsan Mohammadi, Ph.D.
Core Member
Dr. Ehsan Mohammadi’s research focuses on data analytics, online information behavior, scholarly communication, information visualization and health informatics. In particular, he explores how social web data can be useful in shaping the scholarly information ecosystem. Mohammadi is also interested in the information-seeking behaviors of patients and the challenges in online environments to provide appropriate solutions or design useful web applications.
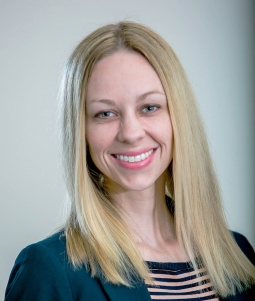
Courtney Monroe, Ph.D., EP-C
Core Member
Dr. Monroe’s research interests center on how to best harness technologies to effectively promote and measure physical activity, as well as to effectively deliver behavioral weight control in adults. She has a particular focus on exploring strategies to best foster social support for these purposes.
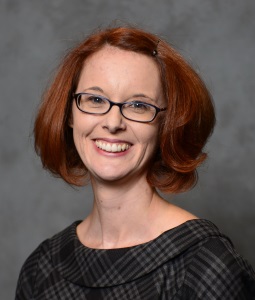
Brie Turner-McGrievy, Ph.D., RD
Core Member
Dr. Turner-McGrievy’s research focuses on discovering ways to help people eat healthier, lose weight, and prevent chronic disease. To achieve this goal, she explores ways to use emerging technology to assist with dietary self-monitoring, physical activity tracking, and provision of social support. In addition, her research focuses on dietary approaches that do not require dietary self-monitoring, such as the vegan and vegetarian diets.
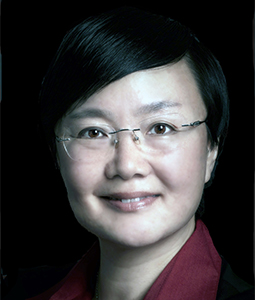
Dezhi Wu, Ph.D.
Core Member
Dr. Dezhi Wu’s research focuses on human-computer/AI interaction, chatbot design, mobile security notification design, health informatics, and social media analytics associated with substance use disorder, vaping, medicine, and prenatal care.